Job Power Profile Prediction and Analysis (2021 - 2023)
Research Staff, July 2021 ~ March 2023
Overview
This project classified HPC jobs based on time-series power consumption to enhance descriptive analytics and train predictive models for data center cooling.
- Developed a machine learning pipeline to classify job power profiles based on consumption patterns.
- Designed a feature extraction framework that distills 186 features from time-series power data.
- Built an open-set neural network classifier to categorize jobs, identifying known and unseen workload patterns.
- Applied generative adversarial networks (GANs) to improve clustering and refine classification models.
- Provided system-wide insights into HPC power usage, informing energy-aware resource management.
Technology
- GAN, k-means, Open-set classification
- Scikit-learn, PyTorch
- Apache Airflow
- OLCF Summit Supercomputer Telemetry
Publication
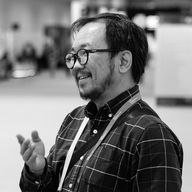